What Can we accept for the future of data analysts? In this blog, I SHARE my own top predictions for 2023.
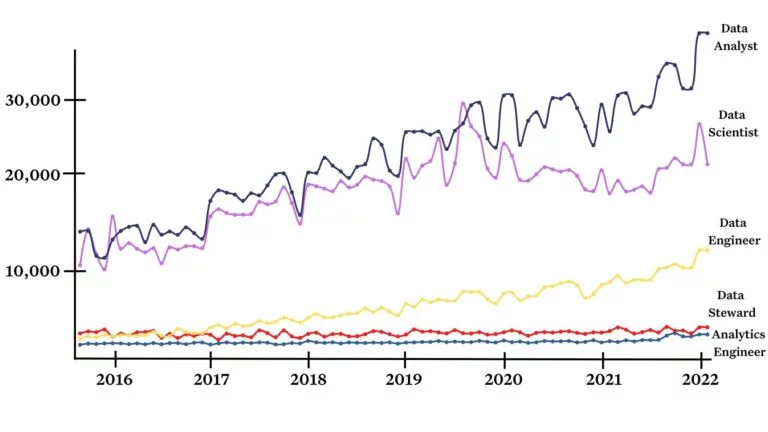
I stumbled across this amazing article shared by Barr Moses, co-founder, and CEO for Monte Carlo, a data consultancy that focuses on data observability. A lot of the insights shared in this article were representative of some substantial changes that are happening inside of my own data team. In my own opinion the Data Analyst role has begun an evolution; combining multiple hats inadvertently pushing the growth of the Analytics Engineer role, as well as the emergence of new roles focused on reliability and observability of data.
As businesses rely increasingly on data to make decisions, the role of data teams is evolving to include not just data analysis, but also ensuring the efficiency, reliability, trustworthiness and ethics of the data being used. With speculation of giant Privacy law reform around the corner in Australia we can expect there to be substantial changes to data governance in Australia within the decade as well. With data breaches and misuse of personal data making headlines, it’s clear that ensuring data trustworthiness and ethics is a major goal for all data teams Australia-wide. Internal stakeholders’ use of data needs to be questioned not just for the sake of ethical use but to understand the use and true requirement of data they need to complete their business objective. We as analysts can generate more value by ensuring we only enable the most efficient use of our data platforms. This provides a secondary benefit of cost-savings on running data platforms by only applying compute power only where it is needed most.
Making sure the data is usefull
A huge step towards driving value for data usage and literacy is to talk to the consumers and business stakeholders to gather requirements. It is essential to frame the conversation in simple business terms and ask relevant questions to understand the use cases, data requirements, and reporting frequency. The article suggests that leaders should engage in this process to shape the conversation, and they can also survey the data consumers en masse. Understanding what data pipelines, models and visualisations are of actual importance to stakeholders and streamlining those data touchpoints by removing unnecessary or redundant models or refactoring inefficient pyspark that could run as effectively with SQL is essential.
Data Trustworthiness: The Key to Data Quality
Data trustworthiness is the assurance that data is accurate, complete, and reliable. Ensuring data trustworthiness is essential for businesses to make informed decisions. However, with data being generated from multiple sources and in different formats, ensuring data trustworthiness can be challenging. Data teams can address this challenge by implementing data quality checks, data validation techniques, and data governance policies.
Data Ethics: The Key to Data Responsibility
Data ethics is the study of the moral principles and values that govern the collection, use, and dissemination of data. With the increasing amount of personal data being collected and analyzed, data ethics is becoming more critical. Data teams can address this issue by implementing data privacy policies, data security measures, and data anonymization techniques. They should also ensure that they are using data in ways that are legal and ethical, and that they are transparent about their data practices.
The Role of Data Teams in Ensuring Data Trustworthiness and Ethics
Data teams will now play a critical role in ensuring data trustworthiness and ethics. They should work closely with other departments, such as legal and compliance, to ensure that they are following best practices and complying with regulations. They should also provide training and education to employees on data trustworthiness and ethics.
Building the ‘Data Mart’
Using dbt and creating a semantic layer can be highly beneficial for data analysts. dbt provides a framework to transform and test data with version control, making it easy to maintain and share. Creating a semantic layer helps analysts to work with clean, structured, and reliable data. It provides a unified view of the data, reduces complexity, and increases consistency in data analysis. A semantic layer also enables analysts to focus on insights and analytics rather than data preparation, allowing them to create better business value. This allows us to create a ‘data mart’ where we can have prebuilt metrics on a linear level that we can pluck from this semantic layer and use for consistent reporting with multiple use cases. Dbt allows us to have observable data lineage for the ‘data mart’ and stakeholder can also be enabled to self-serve from this unified semantic layer.
Conclusion: The Future for Data Analysts
As businesses continue to rely on data to make decisions, the role of data analysts will continue to evolve. Ensuring data trustworthiness and ethics will be the next major crisis for data teams, but by implementing data quality checks, data validation techniques, and data governance policies, they can address this challenge. Data teams will also need to focus on championing efficient data use and optimize their platforms for cost-savings, enabling scale and setting a standard for future efficiency within their respectable data platform.
Update 2025: Everything prediction for the future of data roles I have made in this article have stayed true but for different reasons. With the growth of AI and LLMs data needs to be validated and governed just as effectively but for usage of Artificial Intelligence generated insights. The data role will evolve to be focused towards the possibility of data teams being stewards for AI generated insights and visualisations, ensuring their validity and accuracy and making sure they are not exploited to avoid governance rulings and cause potential data-breaches.